Cohort One
Meet the PhD students who joined the Centre in September 2022! Together they form a cohesive ‘cohort’ of PhD students.
Danny Dixon
Stellar population models predict that the Milky Way should be home to around 100 million stellar black holes – we will never detect the majority of these black holes since they do not emit light on their own. For my PhD, I will develop methods to detect stellar black holes in binaries by observing periodic flares in the luminous companion’s brightness caused by gravitational lensing.
I previously studied theoretical physics at the University of Leeds and data science with astronomy at Durham University before joining the NUdata CDT. With NUdata, I’ve explored statistical learning techniques in various contexts that I hope to apply to detect black holes in upcoming optical surveys.
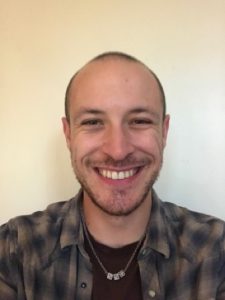
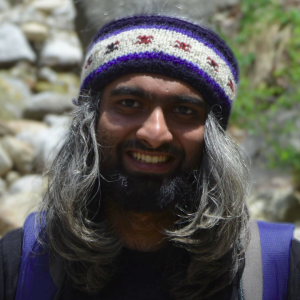
Devang Liya
My PhD project involves studying the cosmic evolution of near-nuclear dust in Active Galactic Nuclei, which are growing supermassive black holes. I use JWST alongside multi-wavelength surveys to study the emission from a large sample of distant AGN. I employ advanced Bayesian techniques to model and decompose this emission, and develop machine-learning techniques to improve existing modelling frameworks.
The NUdata program provides an excellent platform for learning cutting-edge data analysis techniques and building networks with computer scientists — both crucial for my PhD project. Before joining NUdata, I completed my integrated Masters with a physics major and astronomy minor from IISER-Mohali in India.
Harry Birch
My PhD project concerns Solar Prominences and the mechanisms underpinning their structure and evolution. The project will involve the over-11-years-worth of data obtained from NASA’s Atmospheric Imaging Assembly (AIA) onboard the Solar Dynamics Observatory (SDO), using Machine Learning methods to extract properties of prominences and subsequently classify them algorithmically.
It is hoped that through this, an open-source database can be constructed, along with statistical analysis which will help our understanding of the mechanisms surrounding prominence eruptions and solar cycle variations. Outside of my studies I enjoy playing piano and I am a regular badminton player as part of the university society.
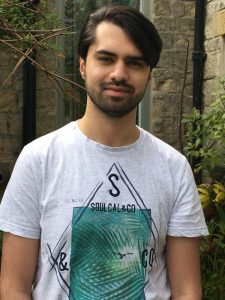
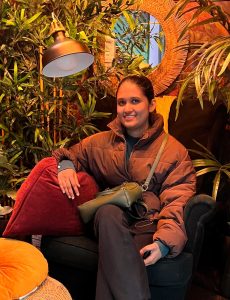
Julie Thomas
I’m a first-year postgraduate research scholar in the School of Mathematics, Statistics and Physics at Newcastle University. My PhD aims to understand the tangling of astrophysical random magnetic fields by using a combination of analytical and numerical approaches, and working at the interface of astrophysics and data science. Random magnetic fields occur in the filaments of the large-scale cosmological structure and affect gas flows in these objects, control thermal conductivity in the collisionless intergalactic plasmas and provide a powerful observational window in the radio range.
The data science training provided as part of NUdata will help me compare high-resolution simulations to large volumes of observational data by using novel statistics and Bayesian approaches to tackle big data problems.
Luke McMullan
As a member of the CDT, I am undertaking a project centered around contributing towards a solution for one of the great mysteries in Solar Physics; the Coronal Heating Problem. Specifically, I am using the skills learnt as a part of the taught components of the CDT program to develop a Machine Learning algorithm for isolating and tracking two key features in the solar atmosphere which have wide ranging impacts on the temperature fluctuations observed in the Corona; namely Coronal Rain, and Nanojets.
Before joining the CDT, I achieved an integrated masters’ at the University of Edinburgh. Here I was inspired to pursue a PhD in Solar Physics when I carried out a project in observing and analysing Solar Spicules in the Chromosphere.
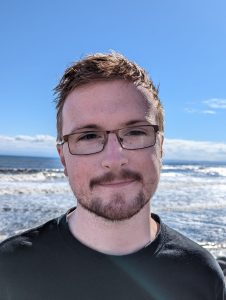
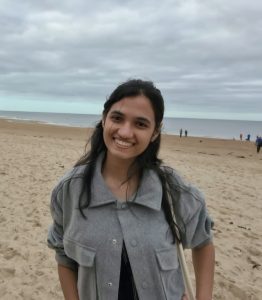
Nikita Balhodi
I am Nikita Balodhi, an NUdata PhD student at Northumbria University, where I work on the applications of deep learning in the field of Solar Physics. I completed my Masters at IISER Tirupati (India), on the detection of barred structures in galaxy images using deep learning.
While traditional Neural Networks are extensively used for solving classification and regression problems in astrophysics, these do not account for any errors in their judgement. These include errors in the estimation of sun’s coronal temperature, its plasma emission measures etc. The goal of my PhD project is to develop Neural Networks that incorporate uncertainty in their predictions through Bayesian approaches and apply them into research areas in Solar Physics.